AI and predictive analytics are rapidly changing the healthcare landscape, offering new ways to improve patient outcomes, streamline operations, and enhance decision-making. As healthcare systems become increasingly data-driven, AI and predictive analytics are at the forefront of driving innovations that improve patient care and make healthcare delivery more efficient and cost-effective. In this article, we will explore the use cases of AI and predictive analytics in healthcare, the best practices for their implementation, and address common challenges that healthcare providers might face.

The global AI in healthcare market size is estimated at around $26.69 billion in 2024.

The global healthcare predictive analytics market size was valued at $11.7 billion in 2022.

The global healthcare IT market size is estimated at around $279.90 billion in 2024.
Introduction to AI and Predictive Analytics in Healthcare
Artificial Intelligence (AI) and predictive analytics are reshaping the healthcare industry by improving patient outcomes and transforming how care is delivered and resources are managed. AI refers to the ability of machines to simulate human intelligence, enabling tasks like data processing, pattern recognition, and decision-making. Predictive analytics, on the other hand, uses statistical algorithms and historical data to predict future outcomes, allowing healthcare providers to make informed decisions.
Leveraging these technologies in healthcare app development enhances diagnostic accuracy, supports personalized treatment plans, and streamlines hospital resource management. By analyzing large datasets, such as patient records and medical images, AI helps detect diseases earlier, while predictive analytics optimizes staffing, equipment maintenance, and overall efficiency.
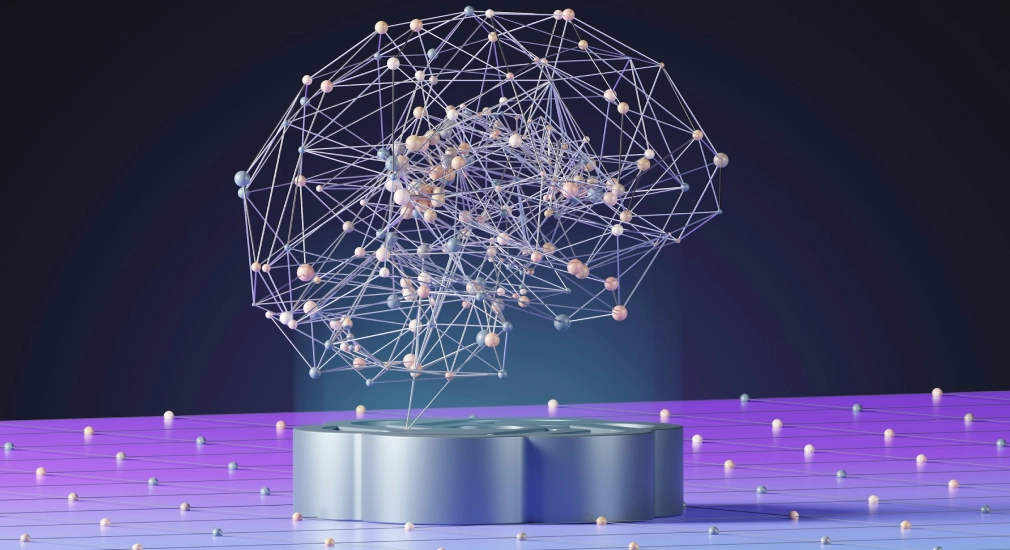
5 Key Use Cases of AI and Predictive Analytics in Healthcare
-
1 Early Disease Detection and Diagnosis
AI’s ability to analyze massive datasets quickly is particularly valuable for early disease detection. Machine learning models can be trained on patient records, medical images, and lab results to identify patterns the human eye might miss. For example, AI systems are being used to detect early signs of cancers, such as breast and lung cancer, from radiological scans. By analyzing patient data and comparing it to historical trends, AI can help predict the onset of diseases like diabetes, Alzheimer’s, and cardiovascular conditions before symptoms become apparent.
One notable example is the use of AI in detecting diabetic retinopathy through retinal imaging. AI algorithms trained on thousands of retinal scans can detect the disease with accuracy comparable to human specialists, potentially saving vision and lives through early intervention.
-
2 Personalized Treatment Plans
One of the most promising applications of AI and predictive analytics is in personalized medicine. Traditionally, treatments have been developed based on what works for the majority of patients, but every individual’s biological makeup is unique. AI allows healthcare providers to analyze patient data, such as genetic profiles, lifestyle habits, and past medical history, to develop personalized treatment plans.
For instance, in oncology, AI-powered predictive models can evaluate a patient’s cancer type, genetic mutations, and response to past treatments to recommend the best course of action. By tailoring treatments to individual patients, healthcare providers can improve treatment outcomes, reduce adverse effects, and provide more efficient care.
-
3 Predictive Maintenance of Medical Equipment
Predictive analytics is not limited to patient care; it also plays a crucial role in managing healthcare resources. Hospitals and healthcare facilities rely on expensive, highly specialized medical equipment like MRI machines and CT scanners. Predictive analytics helps identify potential issues with these machines before they fail. By analyzing data from equipment usage logs and performance metrics, AI can predict when maintenance is needed, preventing costly downtime and ensuring continuous patient care.
For example, hospitals using predictive maintenance systems can schedule repairs before a machine breaks down, ensuring minimal disruption to operations and reducing long-term maintenance costs.
-
4 Hospital Resource Management
In addition to predicting equipment failures, predictive analytics helps hospitals manage their resources more efficiently. Predictive models can forecast patient admissions, emergency room visits, and surgery demands. These predictions enable hospitals to allocate staff, beds, and other resources accordingly, preventing overbooking or understaffing.
AI-powered systems also help optimize supply chain management by predicting the demand for medications and medical supplies. This ensures that hospitals and pharmacies have the necessary inventory without overstocking, reducing waste and cutting costs.
-
5 Predicting Patient Outcomes
Predictive analytics is also being used to forecast patient outcomes. By analyzing patient data and combining it with historical health records, AI can predict which patients are at higher risk for complications, readmission, or mortality. This enables healthcare providers to intervene early, develop preventative strategies, and improve patient outcomes.
For example, AI systems are being used to predict the likelihood of sepsis in hospital patients. Sepsis is a life-threatening condition that can be difficult to detect in the early stages, but predictive models can analyze patient data in real time to alert clinicians of sepsis risks, potentially saving lives.
Schedule a consultation to integrate AI into your healthcare strategy.
Book a callBest Practices for Implementing AI and Predictive Analytics in Healthcare
-
Data Quality and Integration
AI and predictive analytics rely on large amounts of high-quality data. For healthcare organizations, ensuring that their data is clean, accurate, and integrated across different systems is crucial. Electronic health records (EHRs), medical imaging, lab results, and other data sources must be seamlessly integrated to give AI systems a comprehensive view of patient information. Without this integration, the effectiveness of AI models could be compromised.
-
Collaborating with Medical Experts
While AI can process data at scale, medical expertise is essential to validate and interpret the predictions generated by these systems. Collaboration between data scientists, AI specialists, and healthcare providers ensures that AI-driven solutions are clinically relevant and actionable. Along with AI specialists, medical professionals should be involved in every step of AI system development, from defining the problem to evaluating the outcomes.
-
Compliance with Regulations
The healthcare industry is highly regulated, with strict patient privacy and data protection rules, such as the Health Insurance Portability and Accountability Act (HIPAA) in the United States and the General Data Protection Regulation (GDPR) in Europe. Any AI system implemented in healthcare must comply with these regulations to protect patient data. Ensuring that data is anonymized and secure is critical when using AI and predictive analytics in healthcare environments.
-
Staff Training and Adoption
For AI and predictive analytics to be effective, healthcare staff must be trained to use these systems. Training programs should focus not only on technical skills but also on helping staff understand how AI systems can improve patient care. A key factor in AI adoption is fostering trust in these systems by demonstrating their effectiveness and addressing any concerns medical professionals might have about AI replacing human judgment.
-
Continuous Monitoring and Evaluation
Once AI systems are deployed, continuous monitoring and evaluation are essential to ensure that they deliver accurate predictions and align with clinical objectives. Healthcare providers should regularly review AI outputs and adjust the models as needed. Feedback loops between AI developers and healthcare professionals can improve system accuracy and reliability.
Discover how to implement AI into your project.
Contact usChallenges and Ethical Considerations
Despite its potential, the use of AI and predictive analytics in healthcare presents several challenges:
Data Privacy
With AI being used more and more in healthcare, protecting patient data has become a top concern. This means robust data security measures must be in place to prevent data breaches and ensure patient trust.
Bias in Algorithms
AI systems are only as good as the data they are trained on. If the training data is biased, AI algorithms may produce biased results, leading to disparities in healthcare delivery. Ensuring that AI systems are trained on diverse datasets is crucial to preventing bias.
Transparency
Healthcare professionals may be reluctant to trust AI systems if they do not understand how the algorithms arrive at their predictions. Increasing the transparency of AI models and providing explainable AI (XAI) can help foster trust among clinicians.
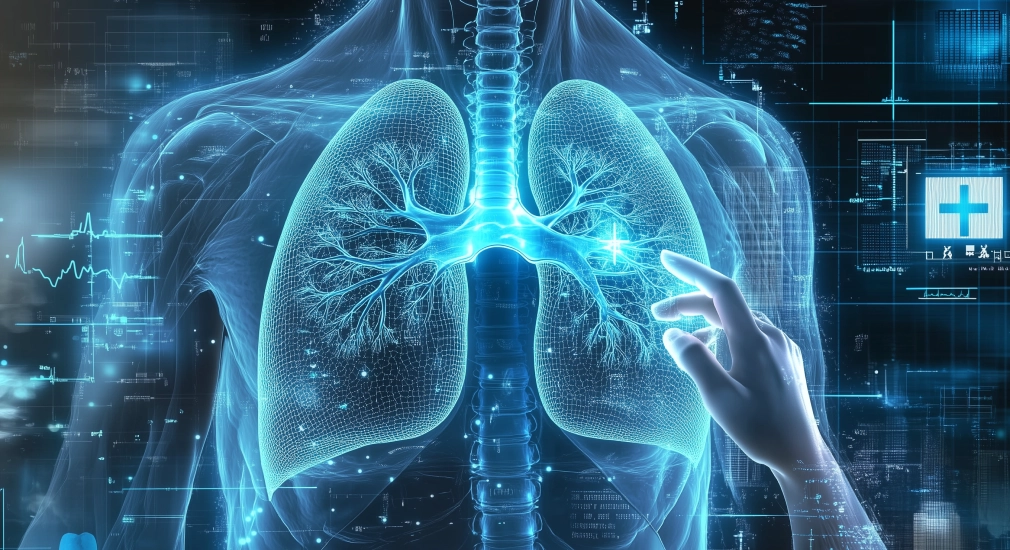
How Can Interexy Help?
Interexy is a leading mobile app development company that stays ahead of the curve with cutting-edge technology solutions. With deep expertise in Artificial Intelligence, Machine Learning, AR/VR, and more, we specialize in creating innovative products across industries like healthcare, FinTech, blockchain, e-commerce, and more.
Our team of skilled developers excels in AI integration, predictive analytics, healthcare IT consulting, and advanced software solutions, ensuring your project is not only brought to life but thrives in today’s competitive landscape. Hire a team of experts within 10 business days for a quick start, ensuring your project moves forward without delay.
Final Thoughts
Artificial Intelligence and predictive analytics offer numerous opportunities to improve healthcare delivery, from early disease detection to optimizing hospital resources. However, successful implementation requires a commitment to best practices, including data quality, collaboration with medical experts, and compliance with regulations. While certain challenges need to be addressed, the potential benefits of AI in healthcare are undeniable.
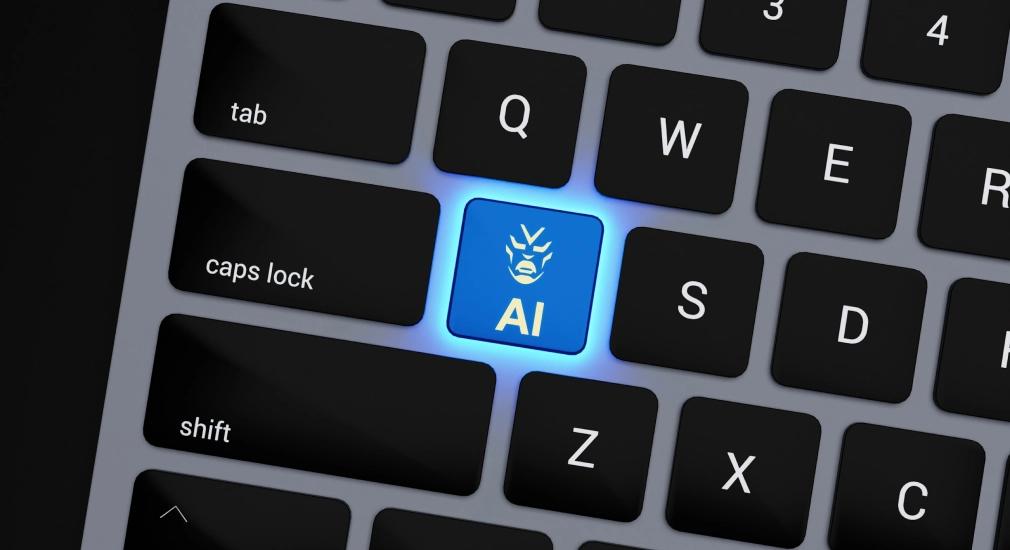
FAQs
-
What is the difference between AI and predictive analytics in healthcare?
AI simulates human intelligence, performing tasks like data processing, pattern recognition, and decision-making. Predictive analytics uses historical data and statistical methods to forecast future outcomes. While AI handles a broader range of tasks, predictive analytics specifically looks at probabilities and trends to support proactive decision-making in healthcare. Both AI and predictive analytics are used together in healthcare to improve patient outcomes.
-
How does AI improve diagnostic accuracy?
AI improves diagnostic accuracy by analyzing vast amounts of medical data, including imaging, lab results, and patient history, at speeds and scales that surpass human ability. This helps medical workers identify patterns and anomalies that may be missed by traditional methods, leading to earlier and more accurate diagnoses.
-
What are the ethical concerns surrounding the use of Artificial Intelligence in healthcare?
Key ethical concerns include data privacy, the risk of algorithmic bias, and the potential for Artificial Intelligence to replace human judgment in clinical decision-making. It’s crucial that AI systems are transparent, secure, and used as a complement to, rather than a replacement for, human expertise.
-
What are the future trends for AI and predictive analytics in healthcare?
Some of the future trends include the increasing use of AI in personalized medicine, where treatments are tailored to individual genetic profiles. Additionally, AI-driven predictive models will play a larger role in preventive care, helping detect diseases before symptoms arise. Integrating AI with wearable technology and telemedicine is also expected to expand, allowing real-time health monitoring and data-driven decision-making for remote care.