Generative AI in Healthcare | Explore the New Revolution in Medical Care and Research
The disparate sources of unstructured data that were prevalent in the medical field and long brought only human errors and challenges now became very useful assets to grow the use of generative AI in the healthcare domain.
The AI technology, as a part of healthtech, relies on Deep Learning algorithms that are able to develop new content, whether in text, audio-visual, code, etc. Generative AI in healthcare can take unstructured datasets and analyze them, which is a breakthrough in the domain because of a large volume of unstructured data such as clinical notes, diagnostic images, medical history, and more.
While AI has already been used in the medical field for some time, generative AI acts as a new tool that helps unlock some of the untapped potential for industry improvement.
Whether used for tedious work and repetitive tasks automation, improving error-prone processes, or modernizing the healthcare system’s infrastructure, generative AI seems to have immense potential. That’s why healthcare executives have to consider and explore the ability to integrate these models into their operational roadmap.

Generative AI in healthcare market size will reach USD 8.7 billion by 2032.

The generative AI market will grow at a CAGR of 35.14% from 2023 to 2032.

98% of healthcare providers say AI boosts enterprise intelligence.
What is Generative AI?
Generative AI is a term used to describe models and algorithms that create brand-new output, including text, photos, videos, code, data, and 3D renderings from data they are trained on. The models generate new pieces of content by referring back to the large volume of data and making new predictions.
The key goal of generative AI is to create content. Other forms of AI are, in contrast, used for different purposes, like analyzing data or helping to control a self-driving car.
Generative AI can also teach machines to learn from provided examples and generate new content according to what they have learned. Its working mechanism is based on complex algorithms that help AI to learn patterns and generate new content similar to the input data.
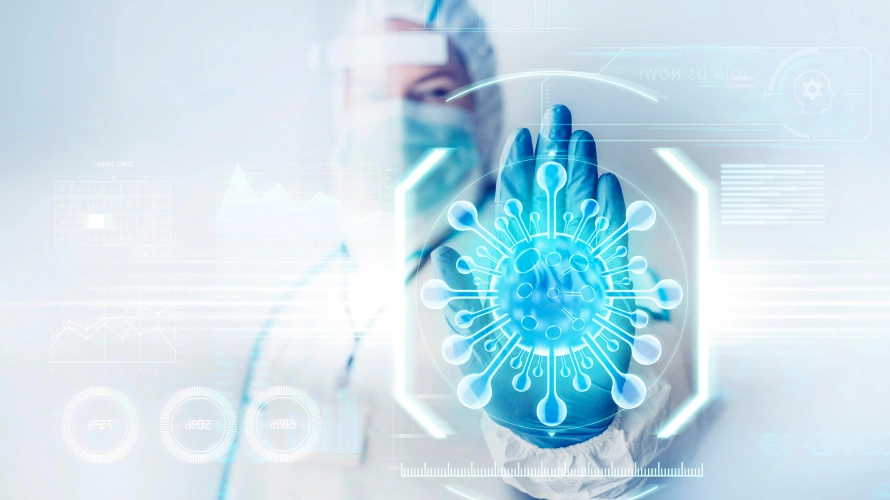
There are two types of generative AI:
Unsupervised Generative AI – this form of AI can learn from unstructured data without any predefined categories and/or labels. Unsupervised generative AI is used when a large volume of data is available, but there is no little or no guidance on how to analyze it.
Supervised Generative AI – this form of AI learns from labeled data. Here, specialists provide AI with input data alongside corresponding labels and/or categories. As a result, AI can learn from this data and then generate new content that will fit into the provided categories.
To wrap the idea up, generative AI is a subset of AI that can teach machines to create something new instead of just analyzing existing data. Generative AI in healthcare mobile app development uses algorithms to generate content such as text, images, and sound. It has many applications, like drug discovery, disease diagnosis, and improved patient care.
How Does Generative AI Work?
Generative AI is working through deep learning neural networks. They are modeled based on the structure of the human brain. These networks are made up of several layers of connected nodes that process input data.
The data is fed into the network, allowing each node to process it and pass it on to the next layer. The female layer then produces the output, which is generated content.
As we mentioned, generative AI can create content in several formats, such as:
- Images;
- Text;
- Sound.
The output will also be based on the input data: text, images, or sound.
7 Use Cases of Generative AI in Healthcare
1. Drug Discovery
It is a common practice for the healthcare domain to take years and even decades to develop medications, mostly because of lengthy and expensive traditional drug discovery procedures.
Considering the need for new drug molecules, generative AI can improve the process by:
- Learning from a large dataset of chemical structures and their attributes, generative AI can generate new molecules analogous to the existing ones. Scientists can then test new molecules and evaluate their potential for further use.
- Identifying suitable candidates and evaluating the efficacy and safety of testing these drugs are the main steps in the time-consuming drug discovery process. Generative AI can speed up and ease the process by identifying possible candidates using a large dataset of drug compounds and their features, matching them with relevant candidates.
- Creating virtual compounds and examining them in silico (a computer simulation rather than a laboratory). Therefore, generative AI can greatly reduce the time and expenses associated with discovering new drugs.
- Designing new molecules that can be used to discover new drugs. The generative AI algorithm learns from a vast repository of chemical structures and their features, creating new molecules tailored for a specific target.
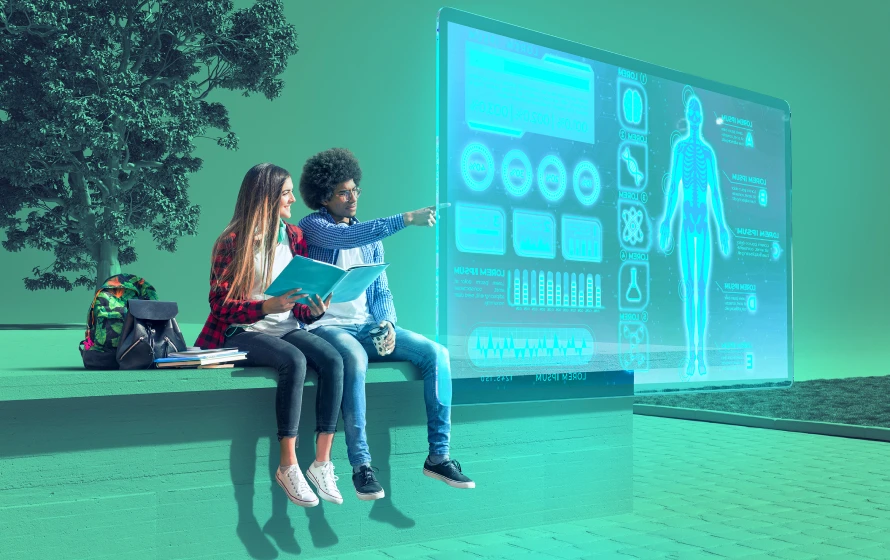
2. Patient Care
Generative AI can create personalized treatment plans. The technology examines a patient’s medical background, genetic data, lifestyle, as well as other crucial factors that help the algorithm develop a highly personalized plan.
For example, the generative AI examines a patient’s tumor DNA and identifies the genetic mutations causing lung cancer. In contrast to existing treatment plans, the algorithm can suggest tailored, accurate plans tackling particular genetic mutations. In addition, generative AI can help doctors predict patient outcomes faster and more precisely, learning from previous similar cases and bringing changes that can help improve chances for new patients.
3. Disease Diagnosis
Generative AI applications have been shown to digitize disease diagnosis by using large volumes of input data, like medical images. They work by identifying patterns associated with specific diseases.
For example, dermatologists can use generative AU’s method to diagnose skin cancer faster. AI here can examine a large volume of skin images to detect patterns suggestive of skin cancer at different stages. Therefore, the doctor can then analyze the suggestions, match them with lab results, and make more diagnoses faster and far more precisely, improving the patient’s chances.
In the same way, generative AI speeds up the diagnosis of conditions by analyzing medical images, like CT scans, X-rays, and MRIs. Following the same procedure, generative AI technology can recognize patterns suggestive of lung cancer.
4. Clinical Documentation
Nuance Communications says that Microsoft is going to support healthcare organizations that use generative AI with its Dragon Ambient eXperience (DAX). This technology has been shown to ease clinical documentation creation and management by capturing and summarizing doctor-patient consultations.
This technology also directly consolidates clinical documentation by recording health data, creating electronic health records (EHR), and easing complex medical language to grow patient’s awareness and understanding.
5. Personalized Medical Chatbots
This is probably one of the most popular generative AI use cases in healthcare and is among the most profitable healthcare app ideas that has already been successfully implemented by some medical providers and institutions. Healthcare organizations of all sizes can develop medical chatbots that will offer patients personalized medical advice and recommendations. For example, Babylon Health built and successfully implemented a generative AI chatbot that asks patients about their symptoms and provides highly personalized medical advice based on input data it was trained on.
6. Medical Imaging
There are some key elements of patient treatment, like MRIs, CT scans, and PET scans, that help swiftly highlight serious injuries and conditions. Generative AI can also boost the process here by offering faster and more accurate imaging diagnoses.
In fact, generative AI in healthcare algorithms can reduce image noise. It can also reduce the time needed for the procedure when coupled with Machine Learning. AI is able to automatically identify different kinds of abnormalities in patient scans without human touch.
7. Medical Research
Speeding up medical research is one of many successful AI use cases in healthcare. In that case, AI can be trained on a large volume of scientific literature that is available online or within a private medical ecosystem, allowing the technology to find patterns related to specific study areas. AI can assist medical researchers in gaining insights and developing new theories.
For example, scientists can open up new pharmacological targets through generative AI. The algorithm finds genes and proteins that are linked to a specific disease by analyzing a large dataset of scientific literature. This can help scientists to find fresh pharmacological targets and create new drugs based on the research.
Make the most of resources, data, and assets within your healthcare organization,
What are the Challenges of Generative AI in Healthcare?
Generative AI in healthcare has many benefits, though it also has several challenges:
-
1. Interpretability
The generated content remains challenging to interpret, so in most cases, it has to be clarified how the algorithm came to a particular result. As a result, there is still a problem for healthcare providers to trust the algorithm and make informed decisions based on its suggestions.
-
2. Need for Large Volume of Data
Generative AI applications require a large volume of data to learn from. In some cases, these datasets might be difficult to obtain or even not exist. This limits the ability of AI to provide valuable insights in certain healthcare areas.
-
3. Higher Transparency
The algorithm could be biased and still tend to produce inaccurate results. While human invention here can resolve the issue, it can still be challenging to identify and address the core issue. This is one of the main reasons for the skepticism among medical providers and patients in terms of AI usage in the healthcare sector.
How Can Interexy Help?
As pioneers in AI healthcare development and implementation, we have a bunch of developers specialized in artificial intelligence in healthcare and machine learning technologies.
Powered by our strong expertise in healthcare data integration, Interexy can get to the nub of the question and resolve issues that you may face associated with AI development and implementation within your medical facilities.
To Wrap it Up
Generative AI has many uses in the medical domain, where drug discovery, disease diagnosis, better patient care, medical imaging, as well as medical research are the key ones.
While there are still some challenges that have to be addressed, a trusted telemedicine development company with strong AI expertise can resolve them and organize smooth AI healthcare software development and implementation.
Book a call with our experts to discuss your case!
Book a call